In April, spring arrived in the valley and filled the woods with bluebells. I joined a local writing group, and went to my first session, as well as attending their monthly spoken word night (the first such event I’ve been to since the pandemic started). I also made a few trips – seeing Helen in Manchester and Muffy in Blackpool, where we visited the tower with its terrifying glass floor. I also saw my family in the Midlands, where I got to meet my neice’s new pigs.
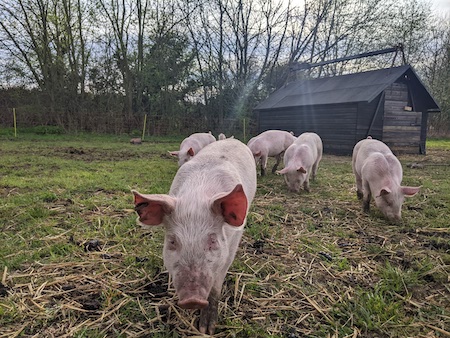
The world outside the valley continues to be a horrorshow. I’m finding the nuclear threats from Russia incredibly disconcerting. Meanwhile coronavirus continues to be an issue, despite the government acting as if it is all over. Two friends have been incredibly ill, one of them ending up in hospital with coronavirus after-effects. I’m risking more events now, but I am still very aware of the ongoing danger.
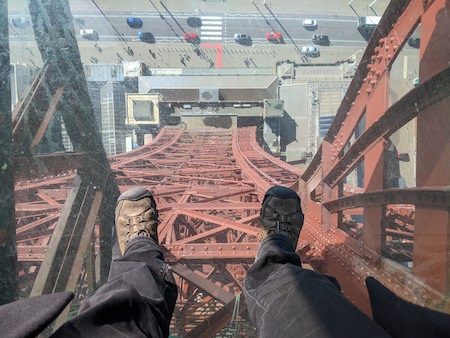
I walked a total of 254,918 steps in April, an average of 8,497 a day, with the maximum being on my visit to Manchester. Now the days are longer I’m going to increase my daily step count. I’ve also been fasting once a week or so, which has lost me a total of 0.9lb over the month. Fast days are, of course, sad and depressing, and I now feel motivated to focus on eating better all round.
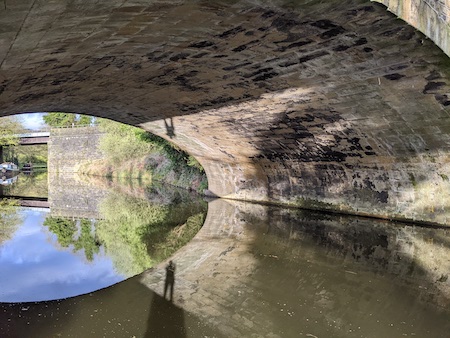
Writing continues to be frustrating. I’m coming to terms with how much I dislike submitting stories (or, indeed, promoting myself very much at all). I’m not sure how to fix that. But I’ve been enjoying writing, particularly since I’ve been having more focussed, flow-based sessons. I was gifted access to Alan Moore’s writing course on BBC Maestro, which has been inspiring. I received some positive validation, with a piece published in the BFS newsletter, No-one knows why they built stonehenge; I also found some etsy reviews I’d missed (“all of the stories in the pamphlets are consistently well-written and simultaneously strange and comforting“). I was delighted to have Dan release of video of him reading my story A Disease of Books.
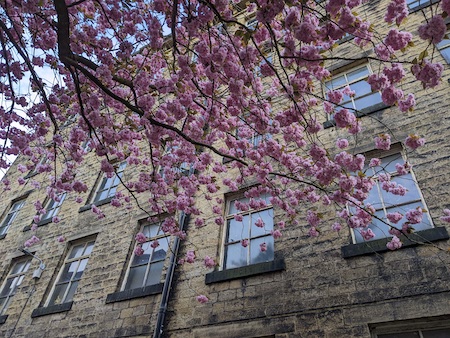
TV has been a mixed bag. I gave up on Moon Knight and Russian Doll‘s second season as I wasn’t enjoying them. Slow Horses was excellent, but I’m a little reluctant to proceed with it as I already have such a strong visual impression of the books that I don’t want to lose. I watched Severance remotely with Kate Shields while she was in covid jail – parts of it were fun, but I found the tension in the season finale contrived. Netflix’s Jimmy Savile documentary A British Horror Story was shocking, and brought the horrors of what happened home in a new way. In the bank holiday at the end of the month I binged the final season of Ozark and was bitterly disappointed by the ending. The conclusion seemed arbitrary and pointless, with little understanding of the story they seemed to have been telling.
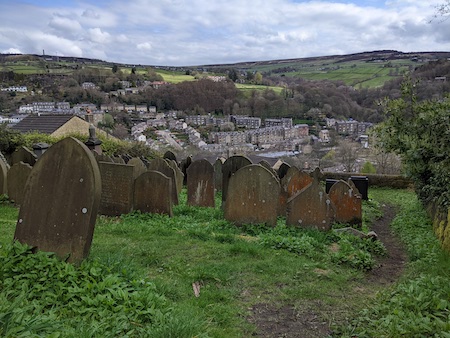
I watched a few movies. Who Killed the KLF was a good retelling of the band’s story. I found Spiderman: Far From Home messy and confusing, but I’m not sure if that was due to the amount of wine drunk beforehand. I rewatched King of New York, a film I’d loved in the 90s and found that it aged reasonably well – and what a cast. A Classic Horror Story was a Netflix recommendation I’d never heard of before but turned out to be an interesting a playful film.
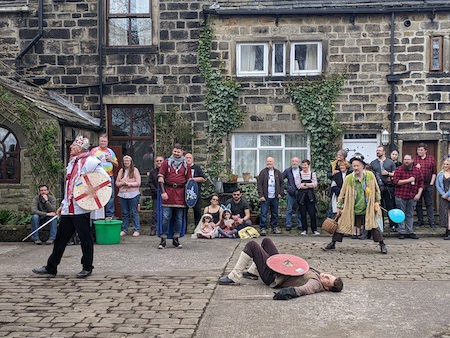
It’s been a good month for books – Emily St. John Mandel’s new book Sea of Tranquility was wise and beautiful, although it suffered a little from being a cover version of a sci-fi classic. While I found the autofiction aspects frustrating, I loved the book’s eerie quality. It’s very much a post-pandemic novel, with some striking observations. Tabitha Lasley’s Sea State was not quite the book about oil working in Scotland that I’d expected, but instead was a raw and vivid account of an affair. I very much enjoyed reading it.
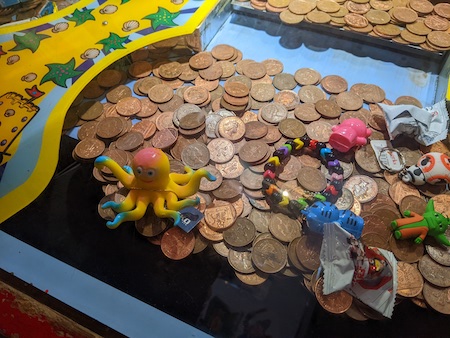
Also good was Until Proven Safe, Geoff Manaugh and Nicola Twilley’s history of quarantine. The book was researched before the pandemic, and looks at some of the huge risks that the world deals with. There are sections on the risks of inter-planetary infection, and the dangers of a plant plandemic. It is a grim book, in one section looking at nuclear materials and the sort of risks that are evaluated here: how an accident with nuclear waste transportation, while unlikely, would result in Las Vegas being abandoned. The book left me aware of how fragile the modern world is.
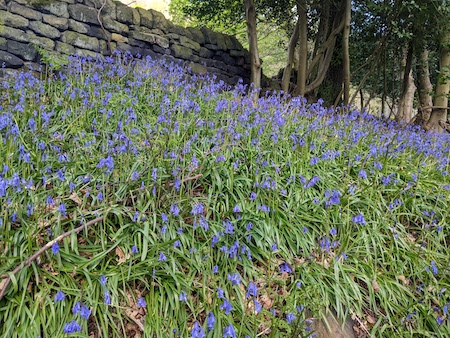
Highlight of the month was Merlin Sheldrake’s Entangled Life, a popular guide to mycology. Every chapter fizzed with ideas and revelations, veering from Terence McKenna (“do psilocybin fungi wear our minds, as Ophiocordyceps and Massospora wear insect bodies?“) to the nature of mycelium networks (“it may be helpful to think of mycelial networks as a type of ‘liquid computer’“). The book was well-referenced, allowing the interesting points to be followed up.
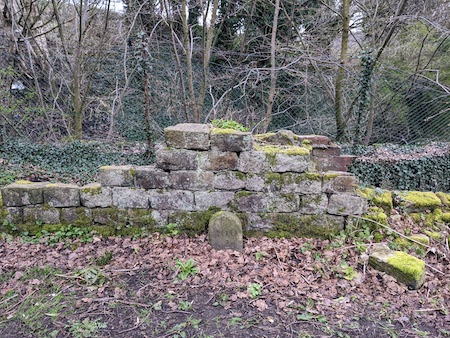